Anomalies of the magnitude of the bias of the maximum likelihood estimator of the regression slope
Citation
O'Driscoll, D., Ramirez, D.E. (2015) ‘Anomalies of the Magnitude of the Bias of the Maximum Likelihood Estimator of the Regression Slope, Essays on Mathematics and Statistics’: Volume 5, Ed. C. Stoica, ATINER, pp.157–166.
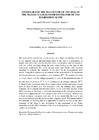
View/ Open
Date
2015Author
O'Driscoll, Diarmuid
Ramirez, Donald E.
Peer Reviewed
YesMetadata
Show full item record
O'Driscoll, D., Ramirez, D.E. (2015) ‘Anomalies of the Magnitude of the Bias of the Maximum Likelihood Estimator of the Regression Slope, Essays on Mathematics and Statistics’: Volume 5, Ed. C. Stoica, ATINER, pp.157–166.
Abstract
The slope of the best-fit line y h x x 0 1 ( ) from minimizing a function of the squared vertical and horizontal errors is the root of a polynomial of degree four which has exactly two real roots, one positive and one negative, with the global minimum being the root corresponding to the sign of the correlation coefficient. We solve second order and fourth order moment
equations to estimate the variances of the errors in the measurement error model. Using these solutions as an estimate of the error ratio in the maximum likelihood estimator, we introduce a new estimator kap 1 . We create a function which relates to the oblique parameter , used in the parameterization of the line from (x,h(x)) to ( ( ), ) 1 h y y , to introduce an oblique estimator lam 1 . A Monte Carlo simulation study shows improvement in bias and mean squared error of each of these two new estimators over the ordinary least squares estimator. In O’Driscoll and Ramirez (2011), it was noted that the bias of the
MLE estimator of the slope is monotone decreasing as the estimated variances error ratio approaches the true variances error ratio. However for a fixed estimated variances error ratio , it was noted that the bias is not monotone decreasing as the true error ratio κ approaches . This paper explains this anomaly by showing that as κ approaches a fixed , the bias of the MLE
estimator of the slope is also dependent on the magnitude of. Other anomalies with the MLE estimator of the slope in the presence of errors in both x and y are discussed.
Keywords
Maximum likelihood estimationMeasurement errors
Moment estimating equations
Oblique estimators